Training Biomedical Relation Extraction Models with a Limited amount of Annotated Data
Reducing the Costs to Extract Medical Knowledge
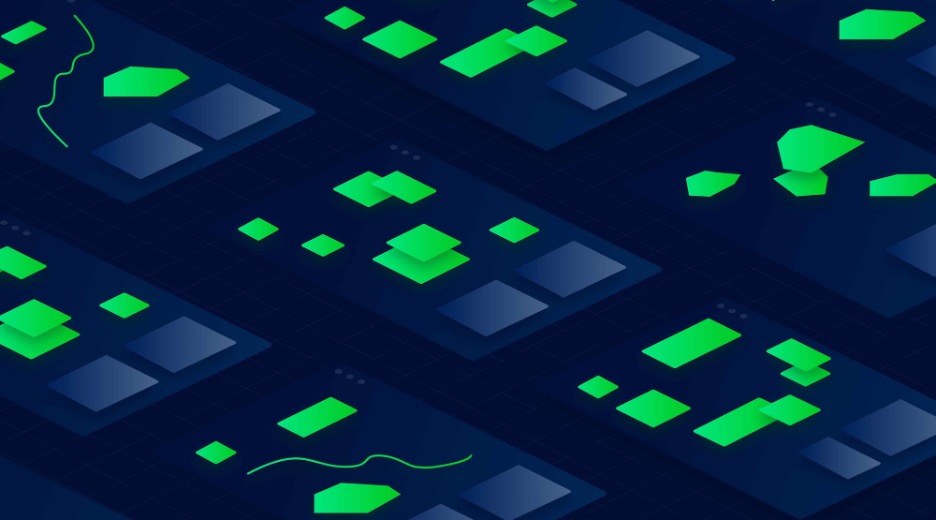
In this blog post we talk about the use of Biomedical Relation Extraction (BioRE) models to speed up and reduce the costs of populating curated databases. How can BioRE models be trained with limited amounts of annotated data? What advantages can such an approach provide? Are there any downsides? Let us find it out!
Populating curated databases
Curated databases are pivotal to the development of biomedical science. However, such databases are usually populated and updated with a great deal of effort by human experts – thus slowing down the biological knowledge discovery process. To overcome this limitation, BioRE aims to shift the population process to machines by developing effective computational tools that automatically extract meaningful facts from the vast unstructured scientific literature. In particular, the discovery of Gene-Disease Associations (GDAs) is one of the most pressing challenges to advance precision medicine and drug discovery, as it helps to understand the genetic causes of diseases.
The costs of training BioRE
Most datasets used to train and evaluate BioRE models are hand-labeled corpora. However, hand-labeling data is an expensive process that requires large amounts of time and, therefore, all these datasets are limited in size.
Distant supervision to the rescue
To reduce hand-labelling requirements, distant supervision has been proposed. Under distant supervision, all the sentences that mention the same pair of entities are labeled by the corresponding relation stored within a reference database. The assumption is that if two entities participate in a relation, at least one sentence mentioning them conveys that relation. As a consequence, distant supervision generates a large number of false positives that can skew BioRE performances. To counter false positives, BioRE under distant supervision can be modeled as a Multiple Instance Learning (MIL) problem. With MIL, the sentences containing two entities connected by a given relation are gathered into bags labeled with such a relation. Grouping sentences into bags reduces noise, as bags are more likely to express a relation than single sentences. Hence, distant supervision alleviates manual annotation efforts, while MIL increases the robustness of BioRE models to noise.So, what are the available datasets that can be used to train BioRE models with limited data? Let us find out!
Distantly supervised datasets
TBGA: TBGA is the first large-scale, semi-automatically annotated dataset for GDA extraction. Overall, TBGA contains over 200,000 instances and 100,000 bags revolving around more than 11,000 genes and 9,000 diseases – obtained from DisGeNET. Besides, compared to current fully distantly supervised datasets, TBGA contains expert-curated data. Hence, TBGA represents a more accurate benchmark than fully distantly supervised datasets.
DTI: DTI is a large-scale, fully-automatically annotated dataset developed to extract Drug-Target Interactions (DTIs). DTI consists of over 600,000 instances and 470,000 bags obtained by aligning drug-target pairs in sentences from nearly 20 million PubMed abstracts against DTI facts from DrugBank.
BioRel: BioRel is a large-scale, fully-automatically annotated dataset developed for general BioRE using UMLS as reference database and Medline as corpus. Overall, BioRel contains over 700,000 instances and 80,000 bags covering more than 120 biomedical relations.
Repositories
So, these were some great BioRE benchmarks!
If you are interested in (any of) them you can also check their data repositories, which are listed below.
– TBGA: click here
– DTI: click here
– BioRel: click here