Publicly available software will include tools for the homogenization of highly heterogeneous images (e.g. compound images), for weakly supervised extraction of multimodal semantic concepts from text and images and to visualize, navigate and refine knowledge structures.
Digital Pathology Transfer Learning
Multiple Instance Learning library
Semi Supervised Learning & Semi-Weakly Supervised Learning implementations
Data driven color augmentation library
Pathology-tiger-baseline
Pathology-tiger-algorithm-example
Pathology-whole-slide-data
Pathology-whole-slide-packer
Pathology-whole-slide-learning
https://www.examode.eu/software/pathology-whole-slide-learning
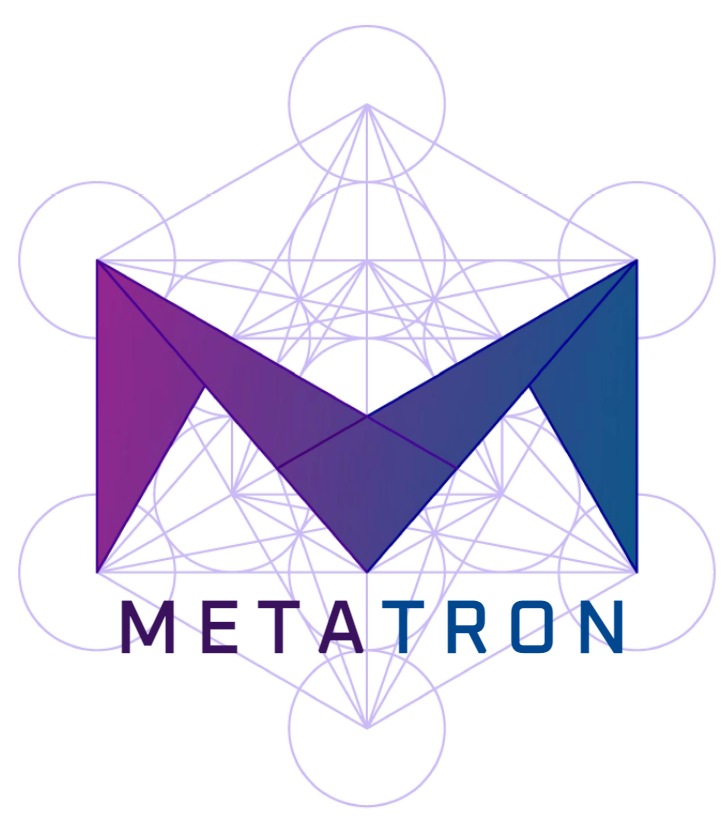
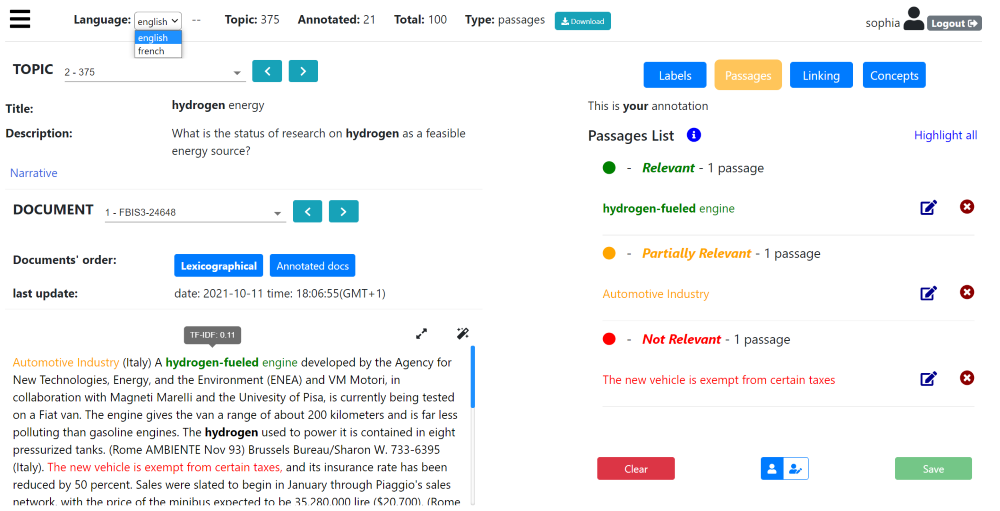
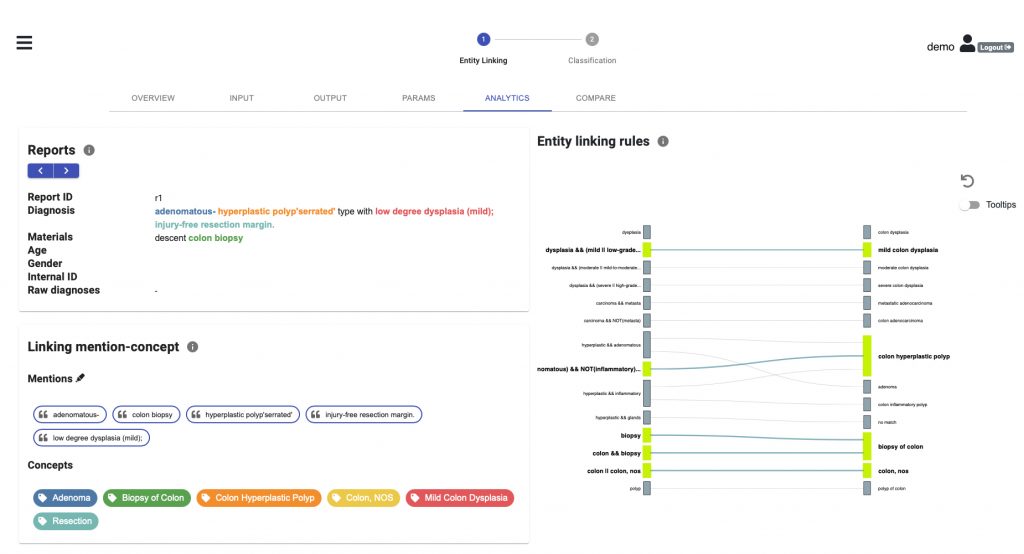
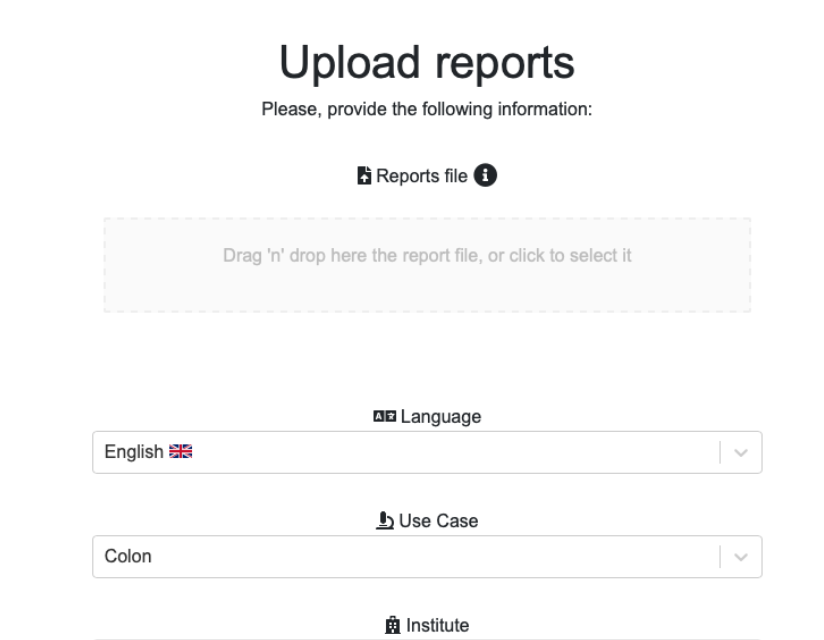
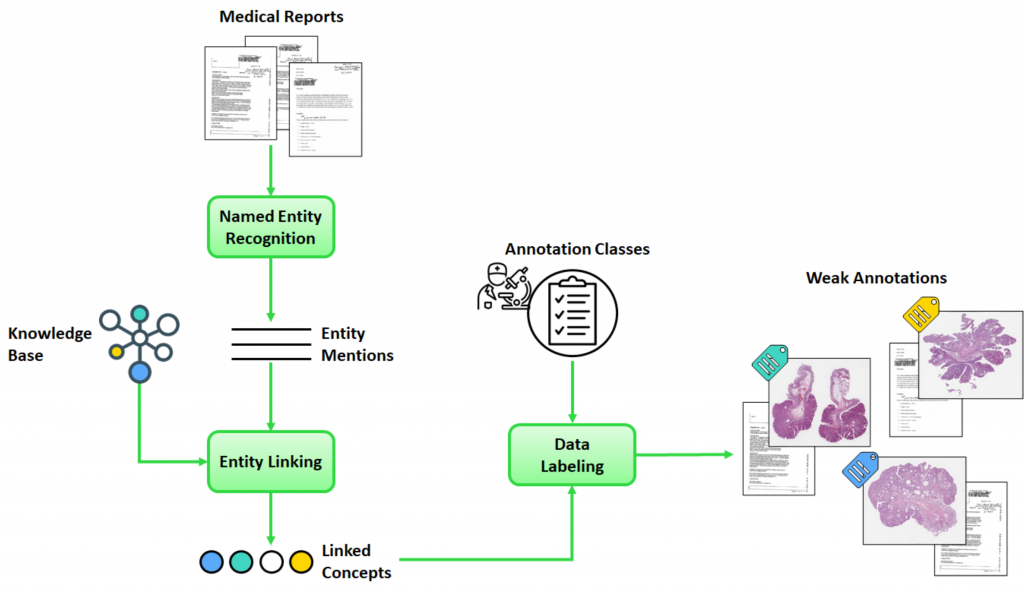
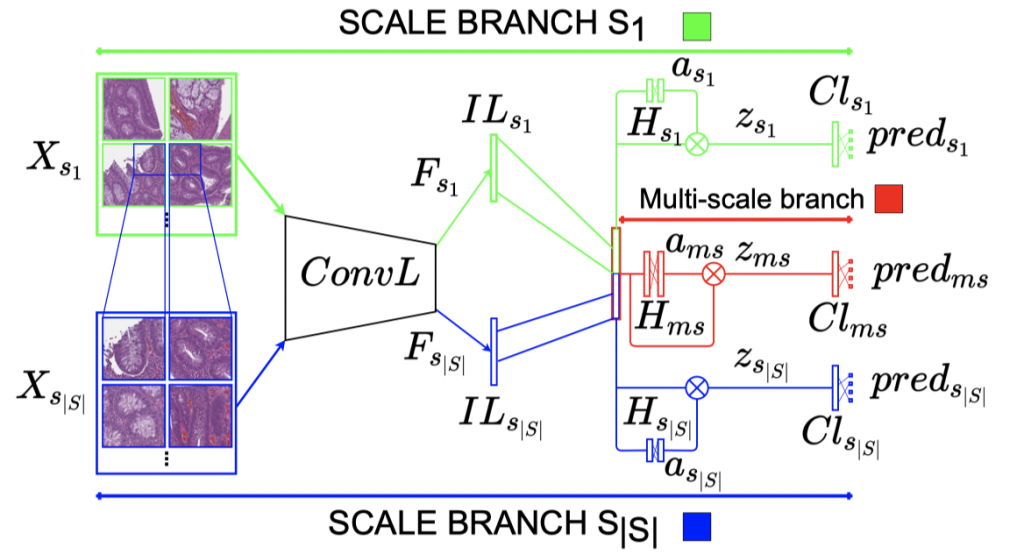
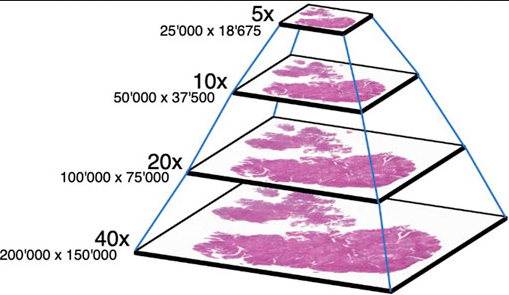
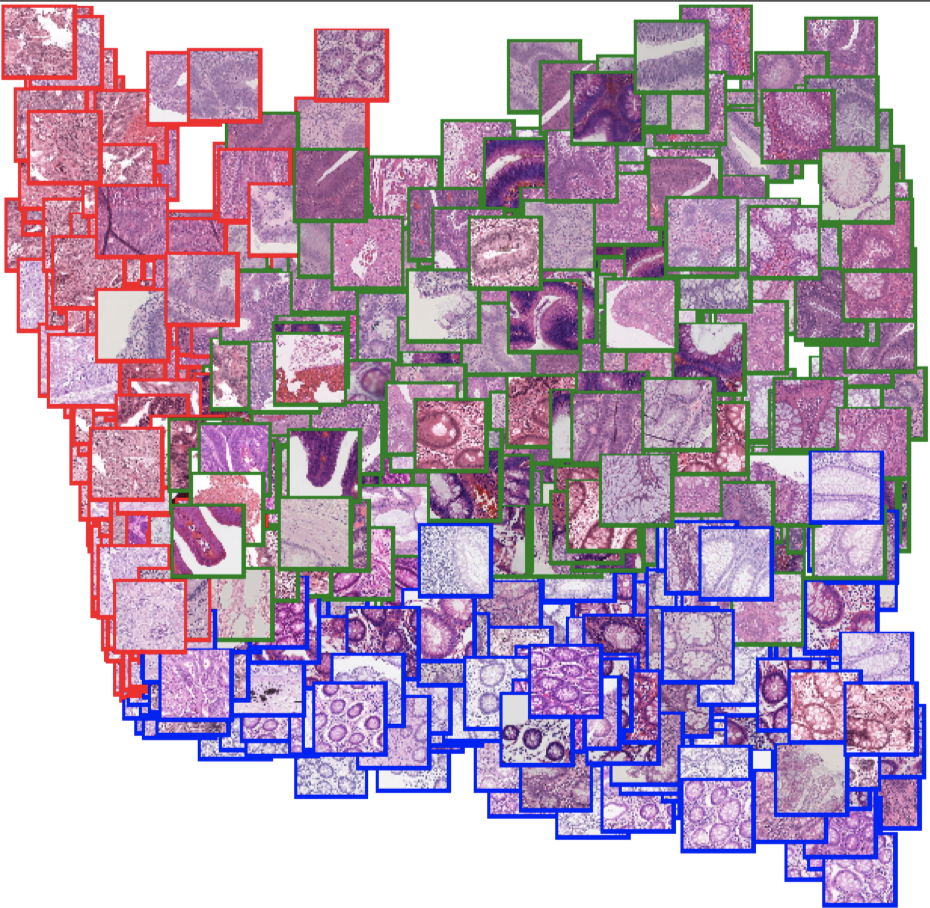
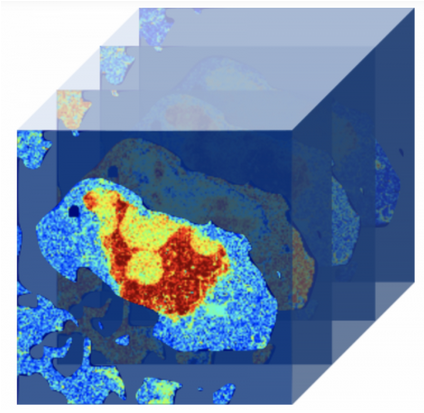
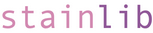
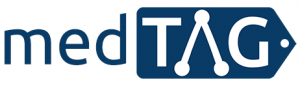
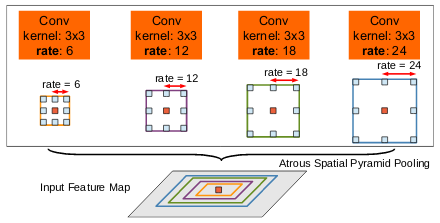